To keep up with the rising popularity of Open AI, Google has released its latest update and progress report for it’s Universal Speech Model (USM). Presently, the USM can handle roughly 300 languages, but the company has set its sights on expanding its capabilities to 1,000 languages.
The development of Google’s Universal Speech Model (USM) marks a crucial initial step towards building artificial intelligence (AI) capable of comprehending and translating 1,000 languages.
Google released groundbreaking details of their AI universal speech model, specially created to comprehend hundreds of spoken languages. The model’s remarkable capabilities are attributed to its extensive training on an impressive 12 million hours of speech and a staggering 28 billion sentences of text, encompassing over 300 languages.
This game-changing technology is poised to transform the landscape of global communication, and the world waits with bated breath for its next move.
The Universal Speech Model (USM) is engineered with a standardised encoding-decoding architecture that utilizes convolutional, attention, and feed-forward modules for its encoder.
This innovative design makes it compatible with LAS, CTC, and RNN-T decoders, pushing the boundaries of what is possible in the realm of artificial intelligence.
Google is leaving no stone unturned in its pursuit of achieving its lofty 1000-language objective. The tech giant is utilising a powerful machine learning model that focuses on the most frequently used languages. Nonetheless, numerous languages have a minimal number of speakers, which restricts the availability of data
In a bid to overcome this hurdle, Google is taking a multi-pronged approach by aggregating and analysing data from various language sources. The goal is to create more accurate results through automatic speech recognition scaling, laying the foundation for a future where language barriers are no longer an obstacle.
According to recent reports, the data available on YouTube is relatively limited in comparison to that of OpenAI. Nevertheless, the Universal Speech Model (USM) has been found to generate 30% fewer word errors than its OpenAI counterpart.
Google says that early tests of the model were designed to create captions on YouTube videos and can perform automatic speech recognition on 100 languages. Some of the languages are spoken by fewer than twenty million people, making it very difficult to find the training data.
This cutting-edge technology marks a significant step forward in the tech giant’s overarching mission to make information easily accessible to everyone, everywhere. With the USM leading the way, the world can look forward to a future where language barriers are no longer a hindrance to communication and understanding.
The machine learning model’s training pipeline is a multi-step process that starts with self-supervised learning of audio files that span numerous languages. Afterward, the quality and language coverage of the data are optimised by incorporating text data wherever possible.
The next step is the fine-tuning of downstream operations, which leverages supervised data to enhance the model’s performance even further. This comprehensive training regimen is a testament to the tech giant’s unwavering commitment to pushing the boundaries of what is possible in the realm of artificial intelligence.
According to a research paper by Google, which was authored by numerous contributors, there are two types of models generated by pre-trained models that can be fine-tuned for downstream tasks. In addition, there are generic automatic speech recognition (ASR) models, which the researchers believe are not fine-tuned downstream.
Google’s researchers assert that these generic ASR models are scalable and can improve the performance of models trained on shorter utterances when applied to longer speech inputs.
Google has also prioritised improving its speech-to-text transcription accuracy as an important area of focus. Specifically, the company has been developing methods to minimise errors and inaccuracies in transcriptions, which can pose significant challenges in certain situations.
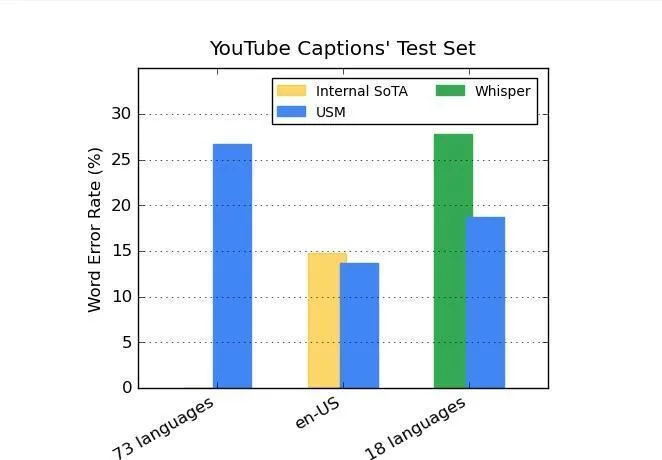
Also, the Google Artificial Intelligence team has been investigating novel approaches to enhance the precision of its speech models by utilising better training data. These endeavors have involved gathering more varied and inclusive datasets, which can aid in improving the model’s capacity to identify and transcribe speech from diverse sources.
In addition, the algorithm is currently confronting several challenges. Based on research and ongoing competitions, the comprehension algorithm must be versatile, robust, and adaptable to facilitate model improvement in a computationally efficient manner, while expanding language coverage and proficiency.
The algorithm should have the ability to process large volumes of data from various sources, generalize to new languages and use cases, and facilitate model upgrades without requiring extensive retraining.
Undoubtedly, there are apprehensions and concerns about the potential misuse or abuse of these technologies. For instance, concerns have been raised regarding the reliability of speech recognition technologies when utilised in legal proceedings or when transcribing discussions that involve delicate or confidential information.
In general, Google’s initiatives in this domain are part of a larger movement aimed at enhancing AI-driven speech recognition and transcription. As voice-activated interfaces become more prevalent, these technologies will assume an increasingly vital function in a wide range of applications, from virtual assistants to customer service chatbots and beyond.
Despite the aforementioned concerns, it is evident that AI-driven speech recognition and transcription will persist as a significant focus for companies like Google in the coming years.
As these technologies continue to advance, they are likely to proliferate and become even more robust, potentially revolutionising our interactions with computers and each other.
Google published a blog entry by members of the team working on the project. It’s team members also published a paper describing the introduction of its Universal Speech Model (USM) on the arXiv pre-print server.