The use of machine learning (ML) has transformed the banking industry in recent years. The banking sector is known for its vast amount of data, and ML has become a valuable tool in analysing this data to gain insights, improve customer experience, and enhance risk management.
The Role of Machine Learning in the Banking Industry
Machine learning is a subfield of artificial intelligence that focuses on developing algorithms that can automatically learn from data. It is an essential tool for analysing large amounts of data quickly and accurately.
The banking sector generates a vast amount of data, including transaction records, customer data, and other financial information. Machine learning algorithms can analyse this data to detect patterns, identify anomalies, and make predictions.
One of the main applications of machine learning in banking is in fraud detection. Fraud is a major problem in the banking industry, and detecting it quickly is essential to prevent financial losses. Machine learning algorithms can analyse transaction data to identify patterns and anomalies that could indicate fraud.
For example, if a credit card is used for multiple transactions in different locations within a short period, the algorithm can flag it as potentially fraudulent. This can help banks to identify and prevent fraud before it occurs.
Another significant application of machine learning in banking is in credit scoring. Banks use credit scores to determine the creditworthiness of applicants. Machine learning algorithms can analyse a wide range of data, including credit history, employment history, and other factors to determine a person’s credit score. This can help banks to make more accurate lending decisions and reduce the risk of default.
Machine learning can also be used to improve customer experience in the banking sector. Banks can use machine learning algorithms to analyse customer data, such as transaction history and browsing behavior, to provide personalised recommendations and offers.
For example, if a customer frequently uses their credit card to purchase airline tickets, the bank could offer them a credit card with air miles as a reward. This can help to improve customer loyalty and retention.
Challenges of Machine Learning in the Banking Sector
Although machine learning has numerous benefits in the banking sector, it also comes with several challenges. One of the primary challenges is the quality of the data. Machine learning algorithms require high-quality data to produce accurate results.
In the banking sector, data can be messy, incomplete, or inconsistent, which can affect the accuracy of the algorithm’s output. Therefore, data cleaning and preparation are critical steps in implementing machine learning in banking.
Another challenge is the lack of transparency in machine learning algorithms. Banks must be able to explain how their algorithms make decisions, especially when those decisions affect customers.
Machine Learning in the Global Banking Market
Globally, machine learning in the banking market was valued at $1.33 billion in 2021 and is projected to reach $21.27 billion by 2031, growing at CARG 32.3% from 2022 to 2023
Machine Learning adoption in the Fintech Market – Statistics
- Banks around the world will be able to reduce costs by 22% by 2030 using artificial intelligence technologies, saving up to $1 trillion, according to a forecast by the research company Autonomous Next.
- It is expected that face recognition technology will increase its annual revenue growth rate by over 20% in 2020, and will be implemented in the banking sector to help prevent credit card fraud.
- According to Insider Intelligence’s AI in Banking report Aggregated cost saving for banks from AI applications is expected to be $447 billion by 2023
Artificial Intelligence (AI) has been making significant strides in the fintech industry
Artificial Intelligence (AI) has been making significant strides in the fintech industry in recent years. Fintech, which stands for financial technology, is an industry that uses technology to provide financial services.
The use of AI in fintech is helping to revolutionise the industry by providing improved efficiency, better customer experience, and enhanced risk management.
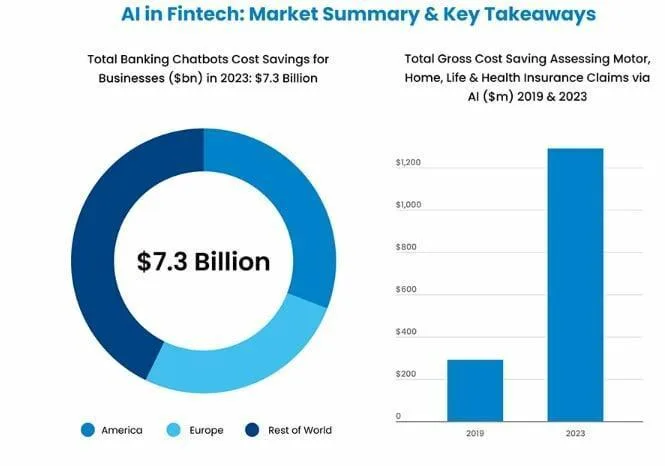
Although AI has numerous benefits in the fintech industry, it also comes with several challenges. One of the primary challenges is the quality of the data.
AI is transforming the fintech industry by providing improved efficiency, better customer experience, and enhanced risk management.
AI in Fintech has numerous applications, including:
- Fraud detection
- Credit scoring
- Improving customer experience
The implementation of AI in fintech comes with several challenges, including the quality of data, transparency of algorithms, and data privacy and security concerns. Therefore, fintech companies must take steps to address these challenges and ensure that their use of AI is ethical and complies with regulatory requirements.
AI and ML capabilities poised to “revolutionise” business processes
According to a Cloudera study, the emergence of data and the tremendous increase in computing power are likely to dramatically change business operations due to artificial intelligence and machine learning.
Nevertheless, many of the companies currently using AI/ML models do not have an appropriate data plan in place. It is becoming increasingly essential to effectively incorporate AI/ML models.
92% of businesses are now leveraging the power of AI/ML, however, more than half are not equipped with a data-based plan to support their use of it.
The authors of the study commented that “dramatic growth in data availability, improved computing power and storage, and more advanced algorithms have made AI/ML a game-changer for businesses”.
Can we trust machine learning in the banking sector?
By using algorithms to analyse large amounts of data, banks can gain insights into customer behavior, detect fraud, and make better lending decisions. However, the question remains: can we trust machine learning in the banking sector, and should we?
One of the main concerns about machine learning in the banking sector is the potential for bias. Machine learning algorithms are only as good as the data that they are trained on, and if that data is biased in some way, then the algorithm will also be biased.
For example, if a bank’s historical data includes a disproportionate number of loans to certain demographics, then a machine learning algorithm may make lending decisions that unfairly disadvantage those demographics.
To prevent this from happening, banks need to ensure that their algorithms are transparent and accountable, and that they are monitoring their algorithms for bias. They also need to be careful about the data that they use to train their algorithms, ensuring that it is diverse and representative of their entire customer base.
Another concern about machine learning in the banking sector is the potential for errors or malfunctions. If an algorithm makes a mistake, it could lead to serious financial consequences for both the bank and its customers. Additionally, if an algorithm is hacked or manipulated, it could be used for fraudulent purposes.
To mitigate these risks, banks need to invest in robust cybersecurity measures and ensure that their algorithms are rigorously tested and validated before being put into production. They also need to have systems in place to monitor their algorithms in real-time and quickly respond to any anomalies or issues.
Some argue that we should be cautious about relying too heavily on machine learning, and instead focus on more traditional approaches to risk management and lending.
Ultimately, the answer to whether we can or should trust machine learning in the banking sector is a nuanced one. While these technologies can provide many benefits, they also come with significant risks that must be carefully managed.
Banks must take a responsible and proactive approach to using machine learning, ensuring that they are transparent, accountable, and focused on delivering value to their customers. By doing so, they can build trust in their use of these powerful technologies and continue to drive innovation in the banking sector.
Deep learning in finance and banking
Deep learning, a subset of machine learning, has rapidly become one of the most important technologies in the finance and banking industries. By analysing vast amounts of data, deep learning algorithms can identify patterns and make predictions, leading to better decision-making and improved outcomes.
One of the most promising applications of deep learning in finance and banking is fraud detection. By analysing transaction data and other sources, deep learning algorithms can identify patterns of fraudulent activity and flag suspicious transactions for further investigation.
Another application of deep learning in finance and banking is in credit risk assessment. By analysing a range of data, including credit history, income, and other factors, deep learning algorithms can predict the likelihood of a borrower defaulting on a loan. This can help financial institutions to make better lending decisions and reduce their overall risk exposure.
In addition, deep learning can be used in finance and banking for customer service and engagement. Chatbots powered by deep learning algorithms can assist customers with basic inquiries and provide personalised recommendations, leading to higher levels of customer satisfaction and loyalty.
Another area where deep learning is making an impact in finance and banking is in asset management. By analysing market trends and other data, deep learning algorithms can identify investment opportunities and predict the future performance of assets. This can help asset managers to make better investment decisions and improve their overall portfolio performance.
Of course, there are also challenges to using deep learning in finance and banking. One of the most significant challenges is the need for large amounts of high-quality data to train these algorithms. Financial institutions need to have robust data management practices in place to ensure that the data they use is accurate and relevant.
Another challenge is the need for technical expertise to develop and deploy these technologies. Financial institutions need to have skilled data scientists and other technical staff on hand to build and maintain these algorithms, which can be costly and time-consuming.
Despite these challenges, however, the potential benefits of deep learning in finance and banking are significant. By leveraging these technologies, financial institutions can improve fraud detection, credit risk assessment, customer service, asset management, and more.
As these technologies continue to evolve, we are likely to see even more innovative applications of deep learning in the finance and banking industries.
Why Effective Digital Banking May Not Require Machine Learning Technology
As digital banking becomes more prevalent, banks are increasingly turning to technology to enhance their security measures. While machine learning (ML) technology is one option, it is important to note that effective digital banking security does not necessarily require ML.
Here are some reasons why:
- Traditional Security Measures Work
Many of the security measures used in digital banking today, such as multi-factor authentication, encryption, and firewalls, have been in use for years and have proven to be effective. While ML can enhance these measures, it is not necessary to replace them entirely.
- ML Can Be Expensive
Implementing ML technology can be costly for banks, both in terms of investment in hardware and software as well as the need for skilled data scientists to develop and maintain the algorithms. For many banks, the cost of implementing ML may not be justifiable.
- ML Is Not Foolproof
While ML can help to identify patterns of fraudulent activity, it is not foolproof. Fraudsters are constantly developing new tactics to evade detection, and ML algorithms may not always be able to keep up. Banks must continue to monitor transactions and develop other security measures to stay ahead of potential fraud.
- Privacy Concerns
ML technology relies on large amounts of data to train the algorithms, which can raise privacy concerns for customers. Banks must be transparent about how they collect and use customer data, and ensure that customer privacy is protected at all times.
- Human Oversight Is Still Important
Even with the most advanced ML technology, human oversight is still necessary to ensure effective digital banking security. Humans can identify patterns of behavior that may be missed by an algorithm, and can make informed decisions based on their experience and expertise.
While ML technology can certainly enhance digital banking security, it is not the only option. By continuing to use traditional security measures, investing in the right hardware and software, and maintaining skilled personnel to monitor transactions, banks can ensure effective digital banking security without relying on ML technology.
Extended Summary
In conclusion, the use of machine learning (ML) in the banking industry has brought about a significant transformation in the way banks operate. ML has brought increased efficiency, accuracy, and speed to various banking operations, ranging from fraud detection and risk management to customer service and engagement.
With ML, financial institutions can leverage vast amounts of data to make more informed decisions, leading to improved business outcomes. The ability of ML algorithms to identify patterns and make predictions based on data has enabled financial institutions to develop new products and services that are better tailored to the needs of their customers.
Despite the benefits of ML in the banking industry, there are also challenges to its adoption. Financial institutions need to have robust data management practices in place to ensure the quality and accuracy of the data used to train ML algorithms. Additionally, financial institutions need to have the technical expertise to develop and deploy these technologies, which can be costly and time-consuming.
Overall, the use of ML in the banking industry is transforming the way banks operate and is enabling financial institutions to provide better services to their customers.
As ML technologies continue to evolve and improve, we can expect even more innovative applications in the banking industry in the future.